AI Integration Gap Analysis Framework for Your Org
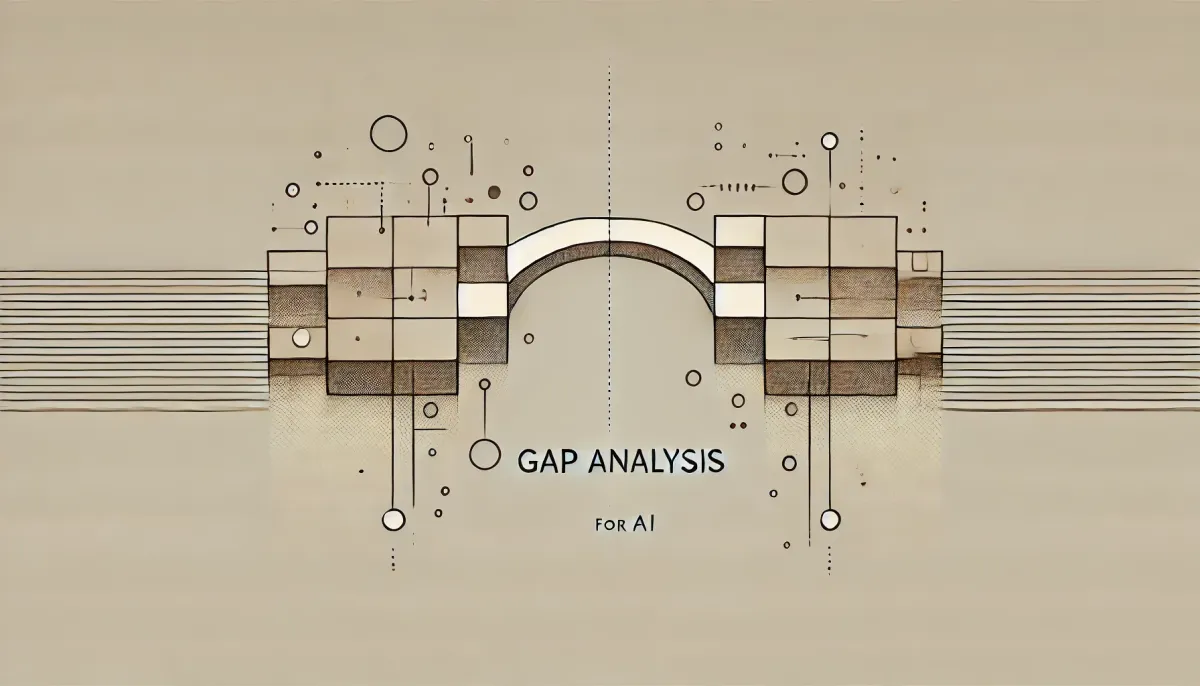
Table of Contents
Introduction
In today's competitive landscape, artificial intelligence represents one of the most significant opportunities for mid-size enterprises to enhance operations, improve customer experiences, and drive innovation. However, the path to effective AI integration is rarely straightforward. This framework gives business leaders a practical approach to assessing their organization's current status, identifying the most valuable AI opportunities, and creating a roadmap for successful implementation.
Unlike typical technology assessments focusing primarily on technical requirements, this framework takes a holistic view that balances business needs, process optimization, data capabilities, technical infrastructure, and organizational readiness. By systematically evaluating these interconnected dimensions, you'll understand where AI can create the most value for your specific business context.
Process Assessment
The foundation of any successful AI initiative begins with a clear understanding of your business processes. Rather than approaching AI as a solution looking for a problem, this assessment helps you identify specific processes where AI can deliver a meaningful business impact.
Begin by mapping your organization's core business processes across all departments. Collect key performance metrics for each process, such as transaction volumes, completion times, error rates, and manual effort requirements. These quantitative measures provide an objective basis for identifying improvement opportunities.
Next, engage with process owners and participants to understand their day-to-day challenges. What bottlenecks do they encounter? Where do they spend disproportionate time on low-value activities? Which decisions require significant judgment but follow consistent patterns? These conversations often reveal hidden opportunities that may not be evident from metrics alone.
With this information, evaluate each process against criteria that indicate AI suitability. Processes that involve repetitive tasks, rule-based decisions, pattern recognition, large data volumes, or natural language understanding typically offer the most significant AI potential. By scoring processes against these criteria, you'll develop a prioritized list of opportunities based on business impact and AI feasibility.
Data Readiness Assessment
AI systems thrive on data, making your organization's data landscape a critical success factor for any AI initiative. This assessment helps you understand whether you have the correct data foundations to support your AI ambitions.
Inventory your existing data sources, including structured databases, document repositories, communication systems, and external data feeds. For each data source, evaluate factors like data volume, update frequency, and ownership. This mapping exercise often reveals both untapped data assets and potential blind spots.
Data quality represents perhaps the most critical factor in AI success. For each key dataset, assess completeness (are all necessary fields populated?), accuracy (how reliable is the information?), consistency (is data standardized across systems?), and timeliness (how current is the information?). AI models trained on poor-quality data will inevitably produce poor-quality outputs.
Beyond the data itself, evaluate how accessible your data is for AI applications. Are systems connected through APIs or other integration mechanisms? Can data flow seamlessly between applications? Are there security or privacy constraints that might limit data usage? These integration considerations often determine how quickly you can move from concept to implementation.
Finally, assess your data governance maturity. Clear ownership, well-defined policies, and established management practices provide the foundation for responsible AI deployment. Without these elements, AI initiatives risk creating compliance issues or eroding trust with customers and employees.
Technical Infrastructure Evaluation
While many AI solutions can be implemented without massive technical overhauls, your existing infrastructure will influence your implementation approach. This assessment helps you identify technical enablers and constraints.
Describe your current computational resources, including on-premise systems, cloud environments, and edge computing capabilities. For each environment, evaluate factors like processing power, storage capacity, and scalability. This inventory helps determine whether you have the necessary foundation for data-intensive AI workloads.
Next, examine your integration architecture. How connected are your systems today? Do you have established APIs and microservices, or are your applications more siloed? The maturity of your integration capabilities often determines how easily AI services can be incorporated into existing workflows.
Evaluate your development and deployment practices as well. Organizations with established CI/CD pipelines, testing frameworks, and monitoring capabilities can typically implement and iterate on AI solutions more quickly than those with more manual processes.
Finally, assess your security and compliance infrastructure. AI implementations often involve sensitive data and mission-critical processes, making security considerations paramount. Document existing security frameworks, identity management systems, and compliance requirements that must be addressed in your AI strategy.
Skill & Capability Assessment
Even the most promising AI technology requires people who can implement, manage, and use it effectively. This assessment helps you understand your organization's human capital readiness for AI adoption.
Start by taking inventory of AI-related knowledge across different levels of the organization. Does your executive team understand AI's capabilities and limitations? Do you have technical staff with data science or machine learning expertise? Are business teams aware of how AI might impact their roles? This knowledge baseline helps identify critical skill gaps and education needs.
Next, evaluate your organization's change readiness based on previous technology adoption experiences. Organizations with strong change management capabilities and cultures that embrace innovation typically achieve faster returns from AI investments.
Assess your learning infrastructure as well. Do you have established training programs, knowledge-sharing mechanisms, and documentation practices that can support adopting new technology? Effective knowledge transfer systems help distribute AI expertise throughout the organization.
Finally, evaluate your ability to acquire and retain AI talent. Given the competitive market for these skills, consider creative approaches, such as partnerships with service providers, internal development programs, or hybrid staffing models, that balance building internal capabilities with external expertise.
Strategic Alignment & Governance
For AI to deliver sustainable value, initiatives must align with strategic objectives and operate within appropriate governance frameworks. This assessment ensures your AI investments support your business strategy and manage associated risks.
Begin by mapping potential AI initiatives to your organization's strategic goals and key performance indicators. This alignment exercise helps prioritize investments and secure executive sponsorship. It also provides a clear framework for measuring success based on business outcomes rather than technical achievements.
Develop a consistent approach to calculating return on investment for AI initiatives. Establishing clear metrics for both costs and benefits creates accountability for results and makes it easier to secure funding for future projects based on demonstrated success.
Evaluate your existing technology governance structures to determine whether they can effectively support AI initiatives. Clear decision-making processes, defined accountability, and appropriate oversight help manage risks without creating unnecessary bureaucracy.
Finally, assess your risk management capabilities regarding AI. Identify potential implementation risks, business continuity considerations, and compliance requirements. Developing mitigation strategies for these risks early in the process prevents costly surprises later.
Implementation Approach
Once assessments across all five domains are completed, you'll have a comprehensive view of your organization's AI readiness and opportunities. The next step is translating these insights into action through a three-phase approach.
During the gap analysis phase, document your current state across all domains and define your desired future based on business objectives. Identify the gaps between these states and prioritize them based on business impact and implementation feasibility.
In the roadmap development phase, define specific initiatives to address prioritized gaps. Sequence these initiatives based on dependencies and impact, allocate resources, establish timelines, and define success metrics. This roadmap provides a structured path forward that balances quick wins with longer-term capability building.
Finally, select and design a pilot project with the highest impact and manageable risk. Define specific objectives, develop a detailed implementation plan, establish baseline metrics for comparison, and create clear criteria for scaling the solution if successful. This pilot-based approach allows you to demonstrate value quickly while learning and adapting before broader deployment.
AI Opportunity Landscape
The assessment process will reveal specific AI opportunities unique to your organization, but several common application areas typically emerge across mid-size enterprises.
In operational excellence, AI can transform process automation through intelligent document processing, quality control, inventory management, and maintenance scheduling. It can also enhance decision support with exception handling prioritization, resource optimization, risk assessment, and compliance monitoring.
For customer experience, AI enables more personalized engagement through conversational interfaces, recommendation engines, journey optimization, and sentiment analysis. It can also improve service delivery through proactive interventions, intelligent case routing, and customer value prediction.
AI provides deeper market intelligence through trend analysis, competitive monitoring, and pricing optimization in product innovation. It can accelerate product development through failure prediction, design optimization, and user feedback analysis.
For workforce enablement, AI amplifies knowledge through expert systems, institutional knowledge capture, and training content generation. It enhances productivity through meeting summarization, priority management, and research acceleration.
Implementing AI in mid-size enterprises doesn't require massive teams or unlimited budgets—it requires a thoughtful, structured approach that balances ambition with pragmatism. By systematically assessing your processes, data, technology, people, and governance, you'll identify the highest-value opportunities for your specific business context and develop a clear path to capturing that value.
The most successful organizations approach AI not as a technology initiative but as a technology-enabled business transformation. They focus on solving real business problems, building on existing strengths, methodically addressing gaps, and continuously learning through measured experimentation.
With this framework as your guide, you can move beyond AI hype to develop a grounded strategy that delivers tangible business value and positions your organization for long-term competitive advantage.